31 Jul 2024
CMU 15-445 notes: Database storage
This is a personal note for the CMU 15-445 L3 notes and CMU 15-445 L4 notes.
1. Data storage
1.1. Volatile device
- The data is lost once the power is off.
- Support fast random access with byte-addressable locations, i.e., can jump to any byte address and access the data.
- A.k.a memory, e.g., DRAM.
1.2. Non-volatile device
- The data is retained after the power is off.
- Block/Page addressable, i.e., in order to read a value at a particular offset, first need to load 4KB page into memory that holds the value.
- Perform better for sequential access, i.e., contiguous chunks.
- A.k.a disk, e.g., SSD (solid-state storage) and HDD (spinning hard drives).
1.3. Storage hierarchy
- Close to CPU: faster, smaller, more expensive.
1.4. Persistent memory
- As fast as DRAM, with the persistence of disk.
- Not in widespread production use.
- A.k.a, non-volatile memory.
1.5. NVM (non-volatile memory express)
- NAND flash drives that connect over an improved hardware interface to allow faster transfer.
2. DBMS architecture
- Primary storage location of the database is on disks.
- The DBMS is responsible for data movement between disk and memory with a buffer pool.
- The data is organized into pages by the storage manager; the first page is the directory page
- To execute the query, the execution engine asks the buffer pool for a page; the buffer pool brings the page to the memory, gives the execution engine the page pointer, and ensures the page is retained in the memory while being executed.
2.1. Why not OS
- The architecture is like virtual memory: a large address space and a place for the OS to bring the pages from the disk.
- The OS way to achieve virtual memory is to use
mmap
to map the contents of a file in a process address space, and the OS is responsible for the data movement. - If
mmap
hits a page fault, the process is blocked; however a DBMS should be able to still process other queries. - A DBMS knows more about the data being processed (the OS cannot decode the file contents) and can do better than OS.
- Can still use some OS operations:
madvise
: tell the OS when DBMS is planning on reading some page.mlock
: tell the OS to not swap ranges outs of disk.msync
: tell the OS to flush memory ranges out to disk, i.e., write.
3. Database pages
- Usually fixed-sized blocks of data.
- Can contain different data types, e.g., tuples, indexes; data of different types are usually not mixed within the same page.
- Some DBMS requires each page is self-contained, i.e., a tuple does not point to another page.
- Each page is given a unique id, which can be mapped to the file path and offset to find the page.
3.1. Hardware page
- The storage that a device guarantees an atomic write, i.e., if the hardware page is 4KB and the DBMS tries to write 4KB to the disk, either all 4KB is written or none is.
- If the database page is larger than the hardware page, the DBMS requires extra measures to ensure the writing atomicity itself.
4. Database heap
- A heap file (e.g., a table) is an unordered collection of pages where tuples are stored in random order.
- To locate a page in a heap file, a DBMS can use either a linked list or a page directory.
- Linked list: the header page holds a pointer to a list of data and free pages; require a sequential scan when finding a specific page.
- Page directory: a DBMS uses special pages to track the location of each data page and the free space in database files.
- All changes to the page directory must be recorded on disk to allow the DBMS to find on restart.
5. Page layout
- Each page includes a header to record the page meta-data, e.g., page size, checksum, version.
- Two main approaches to laying out data in pages: slotted-pages and log-structured.
5.1. Slotted-pages
- The header keeps track of the number of used slots, the offset of the starting of each slot.
- When adding a tuple, the slot array grows from the beginning to the end, the tuple data grows from the end to the beginning; the page is full when they meet.
- Problems associated with this layout are:
- Fragmentation: tuple deletions leave gaps in the pages.
- Inefficient disk I/O: need to fetch the entire block to update a tuple; users could randomly jump to multiple different pages to update a tuple.
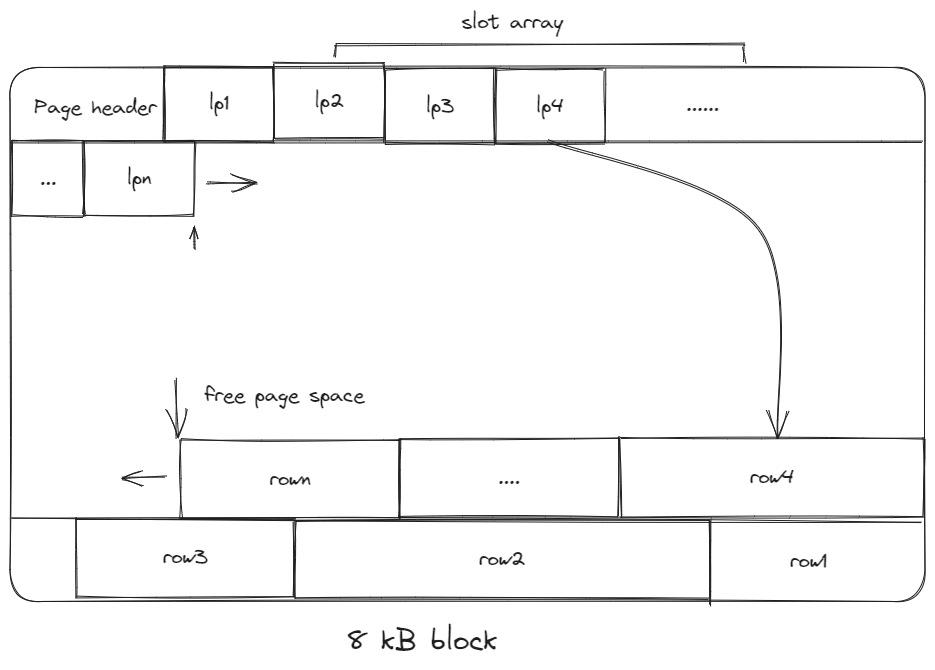
5.2. Log-structured
- Only allows creations of new pages and no overwrites.
- Stores the log records of changes to the tuples; the DBMS appends new log entries to an in-memory buffer without checking previous records -> fast writes.
- Potentially slow reads; can be optimized by bookkeeping the latest write of each tuple.
5.2.1. Log compaction
- Take only the most recent change for each tuple across several pages.
- There is only one entry for each tuple after the compaction, and can be easily sorted by id for faster lookup -> called Sorted String Table (SSTable).
- Universal compaction: any log files can be compacted.
- Level compaction: level 0 (smallest) files can be compacted to created a level 1 file.
- Write amplification issue: for each logical write, there could be multiple physical writes.
5.3. Index-organized storage
- Both page-oriented and log-structured storage rely on additional index to find a tuple since tables are inherently unsorted.
- In an index-organized storage scheme, the DBMS stores tuples as the value of an index data structure.
- E.g., In a B-tree indexed DBMS, the index (i.e., primary keys) are stored as the intermediate nodes, and the data is stored in the leaf nodes.
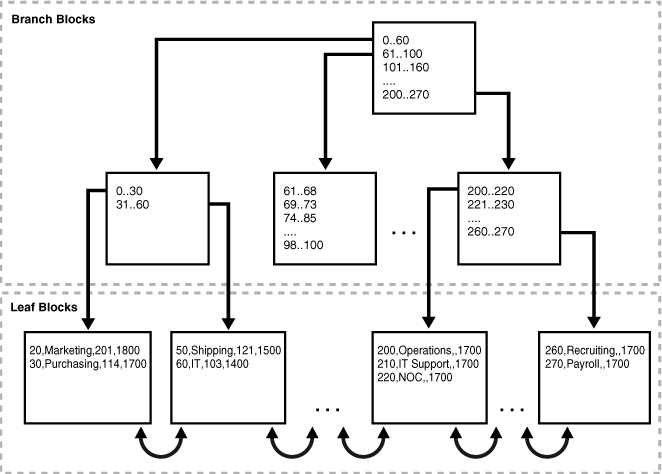
6. Tuple layout
- Tuple: a sequence of bytes for a DBMS to decode.
- Tuple header: contains tuple meta-data, e.g., visibility information (which transactions write the tuple).
- Tuple data: cannot exceed the size of a page.
- Unique id: usually page id + offset/slot; an application cannot rely on it to mean anything.
6.1. Denormalized tuple data
- If two tables are related, a DBMS can “pre-join” them so that the tables are on the same page.
- The read is faster since only one page is required to load, but the write is more expensive since a tuple needs more space (not free lunch in DB system!).
7. Data representation
- A data representation scheme specifies how a DBMS stores the bytes of a tuple.
- Tuples can be word-aligned via padding or attribute reordering to make sure the CPU can access a tuple without unexpected behavior.
- 5 high level data types stored in a tuple: integer, variable-precision numbers, fixed-point precision numbers, variable length values, dates/times.
7.1. Integers
- Fixed length, usually stored using the DBMS native C/C++ types.
- E.g.,
INTEGER
.
7.2. Variable precision numbers
- Inexact, variable-precision numeric types; fast than arbitrary precision numbers.
- Could have rounding errors.
- E.g.,
REAL
.
7.3. Fixed-point precision numbers
- Arbitrary precision data type stored in exact, variable-length binary representation (almost like a string) with additional meta-data (e.g., length, decimal position).
- E.g.,
DECIMAL
.
7.4. Variable-length data
- Represent data of arbitrary length, usually stored with a header to keep the track of the length and the checksum.
- Overflowed data is stored on a special overflow page referenced by the tuple, the overflow page can also contain pointers to next overflow pages.
- Some DBMS allows to store files (e.g., photos) externally, but the DBMS cannot modify them.
- E.g.,
BLOB
.
7.5. Dates/Times
- Usually represented as unit time, e.g., micro/milli-seconds.
- E.g.,
TIMESTAMP
.
7.6. Null
- 3 common approaches to represent nulls:
- Most common: store a bitmap in a centralized header to specify which attributes are null.
- Designate a value, e.g.,
INT32_MIN
. - Not recommended: store a flag per attribute to mark a value is null; may need more bits to ensure word alignment.
8. System catalogs
- A DBMS maintains an internal catalog table for the table meta-data, e.g., tables/columns, user permissions, table statistics.
- Bootstrapped by special code.